Translate this page into:
Leveraging artificial intelligence to promote COVID-19 appropriate behaviour in a healthcare institution from north India: A feasibility study
For correspondence: Dr Madhur Verma, Department of Community Medicine and Family Medicine, All India Institute of Medical Sciences Bathinda, Punjab 151 001, India e-mail: drmadhurverma@gmail.com
-
Received: ,
Accepted: ,
Abstract
Background & Objectives
Non-pharmacological interventions (NPI) were crucial in curbing the initial COVID-19 pandemic waves, but compliance was difficult. The primary aim of this study was to assess the changes in compliance with NPIs in healthcare settings using Artificial intelligence (AI) and examine the barriers and facilitators of using AI systems in healthcare.
Methods
A pre-post-intervention study was conducted in a north-Indian hospital between April and July 2022. YOLO-V5 and 3D Cartesian distance algorithm-based AI modules were used to ascertain compliance through several parameters like confidence threshold, intersection-over-union threshold, image size, distance threshold (6 feet), and 3D Euclidean Distance estimation. Validation was done by evaluating model performance on a labelled test dataset, and accuracy was 91.3 per cent. Interventions included daily sensitization and health education for the hospital staff and visitors, display of information, education and communication (IEC) materials, and administrative surveillance. In-depth interviews were conducted with the stakeholders to assess the feasibility issues. Flagged events during the three phases were compared using One-way ANOVA tests in SPSS.
Results
Higher social distancing (SD) compliance events were flagged by the module in the intervention phase compared to the pre-intervention and post-intervention phases (P<0.05). Mask non-compliance was significantly lower (P <0.05) in the pre-intervention phase and highest in the post-intervention phase, with varied differences between different intervention phases in the registration hall and medicine out-patient department (OPD). The modules’ data safety, transfer, and cost were the most common concerns.
Interpretation & conclusions
AI can supplement our efforts against the pandemic and offer indispensable help with minimal feasibility issues that can be resolved through adequate sensitization and training.
Keywords
Artificial intelligence
compliance
COVID-19
masks
physical distancing
With the pandemic caused by the novel coronavirus (COVID-19), the authorities advised people worldwide to practice various nonpharmacologic interventions (NPIs)1. Due compliance was termed as COVID-19-appropriate behaviour. As per the World Health Organization (WHO), NPIs include all measures or actions, other than the use of vaccines or medicines, implemented to slow the spread of disease in a population2. The NPIs encompass a range of interventions like social distancing (SD), face masks, and hand washing3. Previous studies have demonstrated that among NPIs, SD was more effective in containing COVID-194. However, a lack of spatial awareness can cause unintentional violations of SD. Compliance has been challenging in a densely populated nation like India that witnessed repeated surges in caseload with varied involvement of population groups5.
The novel automated AI systems offer a solution to assess compliance with NPIs without fear of healthcare workers contracting COVID-19 infections. AI has contributed to and helped people maintain NPI compliance in different ways6. Yang et al7 proposed a vision-based real-time system that can detect SD violations and send non-intrusive audio-visual cues using deep-learning models to monitor people’s compliance with the guidelines in public places. A deep learning framework was also proposed to monitor SD via surveillance videography8. In another study, an AI-based facemask-wearing condition identification system was suggested9. MobileNet Mask, yet another deep learning-based model, was proposed to detect faces with or without a mask. Two datasets with over 5200 images were used to train and test the model, which achieved 93 per cent and 100 per cent testing accuracy10. So far, AI has proven reliable and effective in controlling the COVID-19 Pandemic.
Even with so much evidence to support the technique’s usefulness in furthering our efforts towards control of COVID-19 and similar contagious diseases, the use of AI for daily monitoring activities remains unpopular, particularly in resource-constrained countries like India. The pandemic has presented us with a unique situation globally as we continuously look for new and innovative ways to minimise infection rates. AI-based assessment of compliance with NPIs offers an effective solution to enhance COVID-19-appropriate behaviour. With this background, our study assessed the feasibility of using AI to promote COVID-19-appropriate behaviour using the existing resources. The study’s primary aim was to assess the changes in compliance with the most used NPIs (wearing a facemask and ensuring SD) following behavioural change interventions using AI. The objectives were to evaluate and compare the mean compliance with NPI between the baseline, intervention, and post-intervention periods in the OPDs. At the same time, we intended to assess the barriers and facilitators of using AI for surveillance activities.
Materials & Methods
Study design & settings
This pre-post intervention study was conducted at the outpatient department (OPD) of All India Institute of Medical Sciences (AIIMS) Bathinda, Punjab located in north India, after obtaining ethical approval from its Institutional Ethics Committee. The tertiary care institute provides various preventive, promotive, and curative services. The institute has an average daily footfall of around 2000 patients. Due to resource constraints, we installed the AI modules at only three places with maximum footfall: the registration hall, medicine OPD, and Orthopedics OPD areas. Before the interview, the study’s purpose was explained to the stakeholders, and written consent was taken in their preferred language. The manuscript adheres to the STROBE reporting guidelines.
Study duration
The study spanned four months between April and July 2022. The investigation was carried out in three phases: baseline assessment (March 6, 2022 till April 20, 2022; 45 days), intervention phase (April 21, 2022 till May 20, 2022; 30 days), and post-intervention phase (May 21, 2022 till July 4, 2022; 45 days). The baseline and intervention phase witnessed the third phase of the pandemic when OPD services were restricted to only follow up patients and emergencies to minimise hospital-associated transmission of COVID-19. In contrast, the OPD services resumed by the post-intervention period. The hospital started resuming emergency and routine medical and surgical services with their full potential, witnessing a surge in daily visitors to the study site.
Study population
We observed groups of visitors (patients and their relatives accompanying them) to the hospital during OPD hours and assessed their compliance during their stay on the hospital campus.
Study variables
NPIs, like face masks and SD, were our prime dependent variables. Specifically, we did surveillance for less common events, i.e., compliance with SD and non-compliance with facemasks. This is because SD is a dynamic phenomenon, and multiple efforts were being made to ensure SD in different areas for the same person. Hence, compliance was seen as an output. However, compliance with masks was more adopted due to widespread advocacy, and it was a more static process. So, non-compliance was flagged if a facemask was not detected. The study area was our primary independent variable (registration hall, medicine OPD, and Orthopedics OPD)
Study implementation
The study was conducted in the following phases:
A) Model selection
Video recordings from the CCTV cameras pre-installed in the study areas were used, and data were collected for the OPD hours for about 30 days (Box 1; steps 1-8, Fig. 1). The fifth version of the You Only Look Once (YOLO) family of computer vision models was used to assess mask non-compliance, and for SD, 3D Cartesian distance algorithm was deployed additionally, which helped calculate 3D Euclidean distance (straight line distance between the people centroid to quantify the distance), and a threshold of 6ft. (as per COVID social distancing norms) was used to classify if the people were following social distance advisory. Both the models have been previously validated and have satisfactory reliability11,12. Using these algorithms, the final third-party software product (iVizz; not yet available commercially) was developed by experts based in the Indian Institute of Madras Research Park and Silicon Valley, the United States of America, enabling safer healthcare settings by automating infection audits and improving compliance. The software support was provided to the institute free of cost as a part of the company’s Corporate social responsibility.
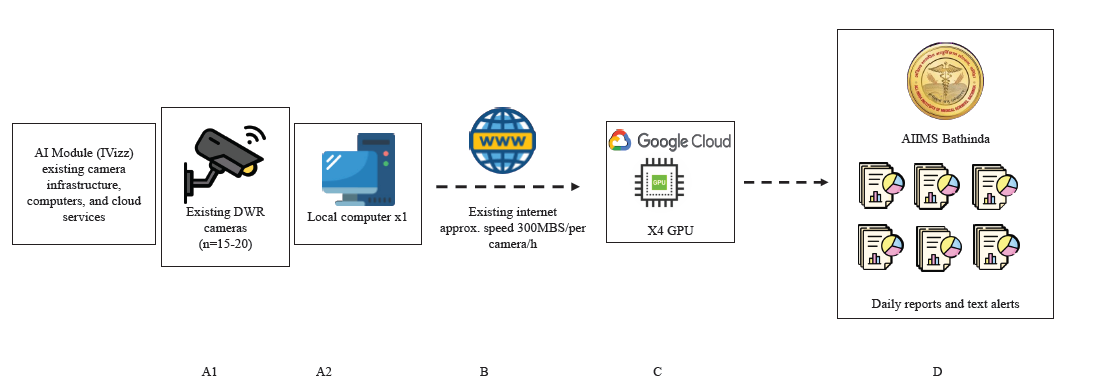
- Flow chart depicting the data assessment process using an artificial intelligence-based software module. Source: Internet copyright free images as declared by the authors.
B) Model training and validation
To reduce bias, approximately 5000 data points were used to train the model with YOLOv5augmentation. Data distribution was done through separate training, validation, and testing processes. Hyperparameters used in the face mask model architecture included confidence threshold (25%), intersection-over-union (IoU) threshold (50%), image size (640), and were trained on 430 epochs with early stopping. For SD, additional parameters like distance threshold (6 feet), 3D Euclidean Distance estimation, and 2D pixel coordinates into real-world (3D) measurements were used. The validation process for the final result involved evaluating its performance on a labelled test dataset for face mask detection and SD compliance. Accuracy was measured by comparing predicted bounding boxes and classes against ground truth labels, with metrics such as mean Average Precision (mAP), precision, recall, and F1-score indicating model effectiveness (91.3%), which was comparable to previous studies from India13.
C) Baseline assessment
Video recordings of the CCTV camera pre-installed in the study areas were used, and data were analysed for the OPD hours every day. The trained model was used to identify compliance with SD and non-compliance with masks by automating data collection using existing video surveillance cameras and improving compliance using a gamification approach. The process is elaborated in the following steps and depicted in a flowchart (Box 1: steps 9-12 and Fig. 1).
D) Intervention phase
In this phase, results from the baseline assessment were circulated among the potential stakeholders, and compliance was ensured by actively using the following interventions that were done biweekly for a month:
i) Sensitization and health education of the hospital staff
The hospital staff, including the health professionals, supporting staff, and security guards, were adequately sensitised regarding the importance of COVID-19-appropriate behaviour and the benefits of the NPI measures.
ii) Administrative surveillance
The Community and Family Medicine department faculties initiated the hospital supervisory rounds to ensure COVID-19-appropriate behaviour in the study areas. If the supervisor observed non-compliant groups, the concerned department faculties and support staff were informed about ascertaining strict compliance. The security guards were directed to pinpoint the patients not following appropriate behaviour at the hospital entrance, and non-compliance restricted their entry into the OPD premises.
iii) Display of IEC materials at prominent places
We displayed standardised IEC material translated into local languages at significant locations to ensure visibility. Short video messages were broadcast on the display screens installed in the waiting hall and OPD areas. Audio messages from the head of the OPD services were also aired regularly to appeal for support in maintaining adequate compliance.
iv) Sensitization of the patients by hospital staff at regular intervals
Patients waiting for their consultations in the waiting hall were made aware of the disease and its preventive measures, with extra emphasis on NPI, with the help of audio-visual aids. They were then motivated to follow SD, use masks, and vaccinate themselves.
E) Post-intervention phase
In this phase, we stopped active interventions and observed compliance due to the residual sensitization of the hospital staff. The data collection approach was similar to the pre-intervention and intervention phases, and compliance was compared between the three steps for meaningful interpretations.
Identification of challenges in implementing NPI using AI
The principal investigator organised weekly meetings with the institution’s co-investigators to discuss the NPI’s progress. Apart from the implementation strategies, various problems encountered during the NPI implementation were discussed, and challenges/feasibility issues were documented in the minutes of the meetings. Possible solutions were also brainstormed.
Statistical analysis
Statistical Package for Social Sciences (SPSS) version 29.0.2.0 (IBM Corp., Armonk, NY, USA) and Microsoft Excel were used for data analysis. Data regarding compliance were made available in the form of absolute numbers. Descriptive statistics and ANOVA were used to compare compliance in different study settings and during the different study periods. Data were represented graphically using line diagrams. Data obtained from the interviews were transcribed into English for the qualitative component. Using grounded theory, we applied thematic analysis of the content to identify the patterns, and two co-authors independently reviewed the paraphrasing and theme assignment.
Results
The study was conducted for four months. The pre-intervention and post-intervention phases were implemented for 45 days each and the intervention phase continued for 30 days (Fig. 2). Overall, there were non-significant differences (P>0.05) in the absolute number of patients visiting the hospital and the two departmental OPDs during the three phases of the study period (Table I).
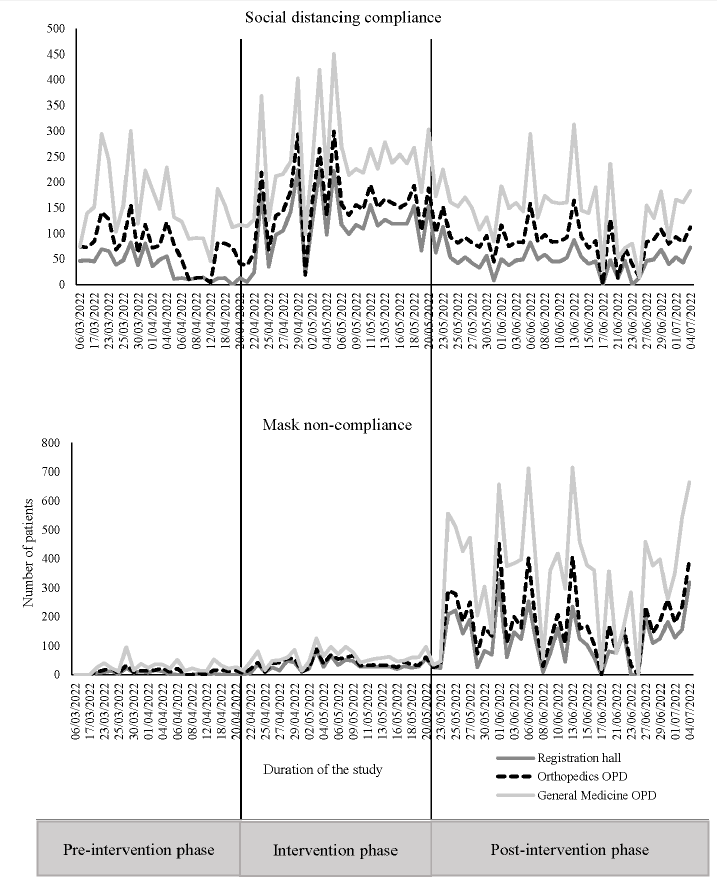
- Trends in SD compliance and face-mask non-compliance during the three phases of the study period in a tertiary care centre in India.
Phase of the study | Per day number of visitors to study sites during the | |||
---|---|---|---|---|
Pre-intervention period | Intervention period | Post-intervention period | P value* | |
Mean±SD (Min-Max) | Mean±SD (Min-Max) | Mean±SD (Min-Max) | ||
Registration hall |
1407.3±259.5 (948-1840) |
1411.4±268 (845-1788) |
1418.7±284.8 (930-1961) |
0.987 |
Orthopaedics OPD |
335.8±117.1 (146-560) |
314.5±103.7 (155-463) |
281±92 (159-466) |
0.14 |
General Medicine OPD |
213.6±30.5 (157-268) |
206.6±34.9 (131-268) |
220.3±42.7 (142-302) |
0.363 |
SD module
There were no outliers, and the data was normally distributed for each group, as assessed by boxplot and Shapiro-Wilk test (P>0.05). Homogeneity of variances was violated for the registration hall and the orthopaedics OPD, as assessed by Levene’s Test of Homogeneity of Variance (P<0.05). Compliance with SD in the registration hall and the medicine OPD was statistically significantly different between different intervention phases. The average number of SD picked up by the AI module was substantially higher in the intervention phase compared to the pre-intervention and post-intervention phases (Table II). Games-Howell post hoc analysis for the registration hall and orthopaedics OPD revealed significant differences in compliance in phase 2 compared to phases 1 and 3. In contrast, non-significant differences were observed between phases 1 and 2. Similarly, Tukey HSD post hoc analysis for the general medicine OPD revealed a significant difference in compliance from phase 2 to phase 3. In contrast, non-significant differences were observed between phases 1 and 2.
Phase of the study | Per day number of visitors highlighted by the AI module in study sites during the | |||
---|---|---|---|---|
Pre-intervention period | Intervention period | Post-intervention period | P value* | |
Mean±SD (Min-Max) | Mean±SD (Min-Max) | Mean±SD (Min-Max) | ||
Compliance to social distancing norms | ||||
Registration hall | 35.3±25.6 (0-82) | 114.1±60.6 (5-227) | 47.0±22.3 (0-113) | <0.001 |
Orthopaedics OPD | 40.1±26.6 (0-74) | 38.8±12.6 (0-77) | 38.8±17.1 (0-80) | >0.05 |
General medicine OPD | 82.4±32.0 (35-152) | 88.7±27.6 (58-155) | 64.3±31.4 (0-149) | 0.006 |
Non-compliance to face mask norms | ||||
Registration hall | 5.2 ±6.4 (0-23) | 30.15±10.9 (0-86) | 121.1±86.1 (0-329) | <0.001 |
Orthopaedics OPD | 5.9±6.2 (0-21) | 5.8 ±6.8 (0-28) | 54.3±38.3 (0-173) | <0.001 |
General medicine OPD | 16.6±14.1 (0-62) | 20.2±11.2 (3-39) | 174.83 ±105.3 (0-311) | <0.001 |
Mask non-compliance module
Like the SD module, the data were normally distributed, as assessed by boxplot and Shapiro-Wilk test (P>0.05). Homogeneity of variances was violated for all three study areas, as assessed by Levene’s Test of Homogeneity of Variance (P≤0.05). Mask Non-compliance was significantly lower in the pre-intervention phase and highest in the post-intervention phase; statistically significantly different between different intervention phases in the registration hall and medicine OPD. Noncompliance was substantially lower in the orthopaedics OPD during the intervention phase compared to the pre-intervention and post-intervention phases. Games-Howell post hoc analysis for the registration hall and medicine OPD revealed significant differences in compliance during the 3 phases. On the other hand, significant differences were only observed between phase 2 and phase 3 of the intervention in the orthopaedics OPD.
Further, the feasibility issues were enlisted after in-depth interviews with the hospital administrators, and their solutions were provided following the discussion with the IT team handling the AI modules (Table III). The modules’ data safety, transfer, and cost were the most common concerns. The other concerns were the accuracy and reliability of the results, utility during rush hours, lack of adequate space in the OPD premises to ensure SD, cost of distributing masks to the patients, and public awareness regarding the pandemic.
Feasibility issues | Possible solutions suggested |
---|---|
The AI modules’ data safety, and data transfer | More clarification by the experts through sessions on methodology in presence of local experts |
Cost of implementing the modules | Initially, the AI module was free as a part of corporate social responsibility, followed by a nominal cost |
The accuracy and reliability of the results | Reliability compared with other known AI modules |
Utility during rush hours | The module improves further through trainings |
Lack of adequate space in the OPD premises to ensure SD | Effective queue management system, and usage of token systems |
Cost of distributing masks to the patients | To be substituted by handkerchief/clothing material bought by the patients |
General public awareness regarding the pandemic | Display of IEC materials at prominent places |
Discussion
The present study used an AI module to evaluate video recordings of the pre-installed CCTV cameras to study the adherence to COVID-19 appropriate behaviour. This was among the few studies from India investigating the feasibility of using AI to assess NPI compliance and exploring the concerns of the potential stakeholders regarding adopting this technique for future preparedness. We report specific exciting findings. First, our AI-based module proved helpful in detecting varied patient behaviours for compliance with SD and masks between different study periods and study areas. Second, we observed marked variations in compliance during and after the study phases. Third, while masks were quickly adopted during pre- and post-study, SD required enforcement, and compliance decreased in the post-intervention period. Lastly, there were varied concerns about using AI among healthcare workers.
Our AI-based modules based on YOLO-V5 and 3D Cartesian algorithms detected varied patient behaviours for compliance with SD and masks between different study periods and study areas. WHO issued advisories in the context of using masks and practising SD to prevent the transmission of COVID-19, which were supported by many studies14,15. Most of the research focused on exploring creative and automatic techniques to detect COVID-19 disease, and very few focused on appropriate behaviour, i.e., using masks and practising SD16,17. The use of AI to detect NPI is a newer concept that has not been explored much. However, more emphasis has been given to studies showing the use of AI-based modules to detect COVID-19-appropriate behaviour during the pandemic. Most of these studies are based on a deep learning-based multi-phase face mask detection model (AI-based Mobile NetMasK)18, Principal Component Analysis based on the classical machine learning method to recognise masked and unmasked faces19, Convolutional Neural Network cameras20, and YOLO models trained with different datasets21,22.
The average number of SD picked up by the AI module was significantly higher in the intervention phase compared to other phases. Stricter implementation of the AI module with adequate sensitization of the supporting staff contributed to improved compliance. In dynamic hospital settings, when new patients report daily, NPI has to be enforced daily. With the rotation of the hospital staff involved in the intervention to other places in the institution as per the rosters, we observed a decrease in compliance in the post-intervention stage. At the same time, increased OPD load in the post-intervention period increased the absolute number of mask non-compliance and decreased SD compliance. Moreover, the public was more compliant with preventive measures in proportion to increased disease severity, which was not the case in the post-intervention phase23.
Our study also observed better face mask compliance than with SD. Mask compliance has been otherwise reported to be low globally but can improve drastically even with a bit of intervention input24. Some studies have depicted better adherence to masks worldwide, but most were based on manual recognition of mask compliance25. AI modules can save numerous human hours exhausted assess compliance. Many countries did not effectively promote mask usage due to a lack of data to support their effectiveness. However, stern advisories from the WHO in support of face masks made the regulations more uniform and acceptable during later phases of the pandemic, as was also seen in our study. With recurring outbreaks despite high vaccination rates, face masks were accepted as a cheaper and non-invasive intervention. On the other hand, compliance with SD has been low in developed and developing countries26. Compliance with SD is related to awareness, as seen in different studies27. Hence, better compliance in our study during the intervention period can be attributed to extensive public awareness campaigns implemented during the intervention. However, compliance slowly waned off in the post-intervention period, highlighting health education’s waning effect28. Attaining adequate compliance is also tricky in settings with high footfall. Hence, we should prefer teleconsultation during peaks of the pandemic, as it can be considerably effective in treating patients and ensuring SD29.
In this study, while the CCTV cameras were already in place, and only a superficial layer was added to analyse the video stream, we observed significant stakeholders’ concerns about deploying AI in healthcare settings. This may be because such augmented capabilities can improve patient care and safety but also lead to an invasive level of scrutiny of the healthcare professionals in their workplace, reducing autonomy and potentially affecting job security or work dynamics. The literature reports such problems under three significant domains: data privacy, ethical and legal30-32. Data privacy was the most critical concern in our study. As the branch is new to most doctors, who are otherwise motivated to use AI in their practice, these concerns restrict its usage. However, the current study uses the encrypted network technology by the Google Cloud platform for data management, which provides multiple levels of complementary defences designed to reduce the risk of configuration errors and attacks. Patient data consists of highly sensitive, personally identifiable information, otherwise protected by the regulation guidelines provided under the Information Technology Act, 2000 (‘the IT Act’), and the Information Technology Rules, 2011, from India. Thus, experts from data sciences and legal matters should be involved in such aspects while making necessary decisions concerning AI. Data leakage was a concern but was dealt with in our study. Healthcare AI adoption needs vast amounts of patient-related data that firms can use for purposes other than intended. The Ministry of Electronics and Information Technology (MeitY) has constituted the Indian Computer Emergency Response Team (‘CERT’), the nodal agency that receives and responds to all breach notifications33. Ethical issues include concerns about Lack of Quality Medical Data, Clinically Irrelevant Performance Metrics, and Methodological Research Flaws32.
Our study had particular strengths and limitations. This is among the few studies from India that have assessed the utility of AI in ensuring COVID-19-appropriate behaviour. We were limited by fewer peer-reviewed studies of AI in healthcare that could have been compared with our study. Most studies have been retrospective and based on historical patient medical records. Nevertheless, the pandemic allowed us to test AI-based interventions in healthcare settings. The major limitation was human detection on the 2D colour images using YOLO v5 for the SD module, which is considered inferior to 3D detection models using more complex AI models but was not possible in the current study due to the lack of computational cost and time availability. However, the two-step algorithm helped us not rely completely on one AI model and provide more acceptable estimates. Further, for mask compliance, the AI model encounters difficulties when faces are positioned at a distance, to the extent that even human annotators may struggle to ascertain whether individuals are wearing masks, resulting in an undetermined label. Enhanced performance from the AI is anticipated with improved data annotation and training over time. Also, mass behaviour is complex and cannot change quickly, so it is challenging to attribute compliance in our study to intervention alone. There was no control group to compare with our intervention arm and assess the effect of interventions in real time. Moreover, the temporal changes in the pandemic and associated guidelines may also have affected compliance; thus, associating the changes only to intervention would be too ambitious.
Specific policy implications and recommendations are emerging in our study. Our study has demonstrated that AI can work in places that are risky for humans, and such advancements can help supplement our routine healthcare activities. However, sustaining such technology with minimal logistics and expertise remains challenging in low-resource countries like India. Moreover, the cost behind such technologies, from development to implementation, must be considered. The government has already spent a lot on managing the pandemic, and we should think of more feasible and cost-effective interventions to control the spread of infection. The scope of using AI is enormous and can extend beyond COVID-19 to further our efforts against tuberculosis and similar illnesses. AI can immensely help improve hospital hygiene in ICUs and laboratories. We should also offer more awareness programmes to acquaint users with emerging technologies. Policymakers should consider including AI lectures in the medical curriculum, which would immensely help the medical fraternity.
To conclude, the AI module has shown much potential in monitoring SD and mask compliance during a limited study time. Through our fundamental analysis, we could show compliance changes backed up by trivial enforcements and awareness drives. The same strategies can be used in other public places to supplement the government’s efforts in pandemic management. While there were concerns about using AI in health, such as data security and ethical issues, they could be resolved. SD and mask compliance depend on many factors active at personal, societal, environmental, and policy levels that need to be studied further to help us understand the actual reasons for non-compliance and further our efforts against the pandemic.
Acknowledgment
Authors are thankful to Mr. Arnav Goel, PhD scholar, Geospatial Data Science, Purdue University, who helped us immensely by providing critical insights regarding artificial intelligence and improving the current version of the manuscript.
Financial support & sponsorship
None.
Conflicts of Interest
None.
Use of Artificial Intelligence (AI)-Assisted Technology for manuscript preparation
The authors confirm that there was no use of AI-assisted technology for assisting in the writing of the manuscript and no images were manipulated using AI.
References
- Does oxford government response tracker explain the SARS-CoV-2 morbidity and mortality: An ecological time series study. JMIR Public Health Surveill. 2023;9:e38371.
- [CrossRef] [PubMed] [PubMed Central] [Google Scholar]
- Non-pharmaceutical public health measures for mitigating the risk and impact of epidemic and pandemic influenza. Available from: https://www.who.int/publications/i/item/non-pharmaceutical-public-health-measuresfor-mitigating-the-risk-and-impact-of-epidemic-and-pandemic-influenza, accessed on January 16, 2024.
- Personal prophylaxis against COVID-19: A compilation of evidence based recommendations. J Pak Med Assoc. 2020;70:S15-S20.
- [Google Scholar]
- Exploring the utility of google mobility data during the COVID-19 pandemic in India: Digital epidemiological analysis. JMIR Public Health Surveill. 2021;7:e29957.
- [CrossRef] [PubMed] [PubMed Central] [Google Scholar]
- Prevalence of depression, anxiety, and insomnia among healthcare workers during the COVID-19 pandemic: A systematic review and meta-analysis. Brain Behav Immun. 2020;88:901-7.
- [CrossRef] [PubMed] [PubMed Central] [Google Scholar]
- Applications of robotics, artificial intelligence, and digital technologies during COVID-19: A review. Disaster Med Public Health Prep. 2022;16:1634-44.
- [CrossRef] [PubMed] [PubMed Central] [Google Scholar]
- A vision-based social distancing and critical density detection system for COVID-19. Sensors (Basel). 2021;21:4608.
- [CrossRef] [PubMed] [PubMed Central] [Google Scholar]
- Monitoring COVID-19 social distancing with person detection and tracking via fine-tuned YOLO v3 and Deepsort techniques. Available from: https://arxiv.org/abs/2005.01385v4, accessed on January 16, 2023.
- Identifying facemask-wearing condition using image super-resolution with classification network to prevent COVID-19. Sensors (Basel). 2020;20:5236.
- [CrossRef] [PubMed] [PubMed Central] [Google Scholar]
- MobileNet mask: A multi-phase face mask detection model to prevent person-to-person transmission of SARS-CoV-2. In: Kaiser MS, Bandyopadhyay A, Mahmud M, Ray K, eds. Proceedings of International Conference on Trends in Computational and Cognitive Engineering. Advances in Intelligent Systems and Computing. Vol 1309. Singapore: Springer; Available from: https://doi.org/10.1007/978-981-33-4673-4_49.
- [Google Scholar]
- Research on mask-wearing detection algorithm based on improved YOLOv5. Sensors (Basel). 2022;22:4933.
- [CrossRef] [PubMed] [PubMed Central] [Google Scholar]
- Deep visual social distancing monitoring to combat COVID-19: A comprehensive survey. Sustain Cities Soc. 2022;85:104064.
- [CrossRef] [PubMed] [PubMed Central] [Google Scholar]
- Face mask detection using deep learning: An approach to reduce risk of Coronavirus spread. J Biomed Inform. 2021;120:103848.
- [CrossRef] [PubMed] [PubMed Central] [Google Scholar]
- Effectiveness of face masks in preventing airborne transmission of SARS-CoV-2. mSphere. 2020;5:e00637-20.
- [CrossRef] [PubMed] [PubMed Central] [Google Scholar]
- Transmission of COVID-19 virus by droplets and aerosols: A critical review on the unresolved dichotomy. Environ Res. 2020;188:109819.
- [CrossRef] [PubMed] [PubMed Central] [Google Scholar]
- Detection methods of COVID-19. SLAS Technol. 2020;25:566-72.
- [CrossRef] [PubMed] [PubMed Central] [Google Scholar]
- Automatic segmentation of MR brain images with a convolutional neural network. IEEE Trans Med Imaging. 2016;35:1252-61.
- [CrossRef] [PubMed] [Google Scholar]
- Real-time implementation of AI-based face mask detection and social distancing measuring system for COVID-19 prevention. Sci Program. 2021;2021:1-21.
- [CrossRef] [Google Scholar]
- Implementation of principal component analysis on masked and non-masked face recognition. In: 2019 1st International Conference on Advances in Science, Engineering and Robotics Technology (ICASERT). IEEE; 2019. p. :1-5.
- [Google Scholar]
- A novel GAN-based network for unmasking of masked face. IEEE Access. 2020;8:44276-87.
- [CrossRef] [Google Scholar]
- Fighting against COVID-19: A novel deep learning model based on YOLO-v2 with ResNet-50 for medical face mask detection. Sustain Cities Soc. 2021;65:102600.
- [CrossRef] [PubMed] [PubMed Central] [Google Scholar]
- Social distancing detector using deep learning. Int J Recent Technol Eng. 2022;10:146-9.
- [CrossRef] [Google Scholar]
- Perceptions towards COVID-19 and adoption of preventive measures among the public in Saudi Arabia: Across sectional study. BMC Public Health. 2021;21:1251.
- [CrossRef] [PubMed] [PubMed Central] [Google Scholar]
- Impact of community masking on COVID-19: A cluster-randomized trial in Bangladesh. Science. 2022;375:eabi9069.
- [CrossRef] [PubMed] [PubMed Central] [Google Scholar]
- Increasing face-mask compliance among healthcare personnel during the coronavirus disease 2019 (COVID-19) pandemic. Infect Control Hosp Epidemiol. 2022;43:616-22.
- [CrossRef] [PubMed] [PubMed Central] [Google Scholar]
- Non-compliance to social distancing during COVID-19 pandemic: A comparative cross-sectional study between the developed and developing countries. J Public Health Res. 2021;11:2614.
- [CrossRef] [PubMed] [PubMed Central] [Google Scholar]
- Study on awareness of COVID-19 and compliance with social distancing during COVID-19 pandemic in Indonesia. J Community Psychol. 2022;50:1564-78.
- [CrossRef] [PubMed] [PubMed Central] [Google Scholar]
- Lockdown efficacy in controlling the spread of COVID-19 may be waning due to decline in public compliance, especially among unvaccinated individuals: A cross-sectional study in Israel. Int J Environ Res Public Health. 2022;19:4943.
- [CrossRef] [PubMed] [PubMed Central] [Google Scholar]
- Client satisfaction with telemedicine services during COVID-19 pandemic: A cross-sectional survey from a teaching institute of North India. J Family Med Prim Care. 2022;11:5187-93.
- [CrossRef] [PubMed] [PubMed Central] [Google Scholar]
- Privacy protection in using artificial intelligence for healthcare: Chinese regulation in comparative perspective. Healthcare (Basel). 2022;10:1878.
- [CrossRef] [PubMed] [PubMed Central] [Google Scholar]
- Legal concerns in health-related artificial intelligence: A scoping review protocol. Syst Rev. 2022;11:123.
- [CrossRef] [PubMed] [PubMed Central] [Google Scholar]
- Ethical concerns around use of artificial intelligence in health care research from the perspective of patients with meningioma, caregivers and health care providers: A qualitative study. CMAJ Open. 2020;8:E90-E95.
- [CrossRef] [PubMed] [PubMed Central] [Google Scholar]
- Indian computer emergency response team. Available from: https://www.cert-in.org.in/, accessed on March 31, 2023.