Translate this page into:
Piloting an event-based surveillance model in private hospitals for early detection of disease clusters, Kerala, India
For correspondence: Dr Manoj V. Murhekar, ICMR-National Institute of Epidemiology, Chennai 600 077, India e-mail: mmurhekar@gmail.com
-
Received: ,
Accepted: ,
Abstract
Background & objectives
Event-based surveillance (EBS) is a critical component of early warning systems for detecting and responding to infectious disease outbreaks. While EBS is widely used in public health settings, its integration into private healthcare facilities remains limited. This study undertook to pilot an EBS in private hospitals in Kasaragod, Kerala and to assess its added value in early detection of disease clusters.
Methods
Clinical nurses abstracted the data on hospitalisation dates, places of residence, and presenting illnesses from case records of patients with acute febrile illness (AFI) admitted in six private hospitals. A software algorithm analysed the data to identify spatiotemporal clustering of case-patients or deaths (signals), for syndromes of interest [acute febrile illness with rash (AFIR), acute encephalitis syndrome (AES), acute febrile illness with haemorrhage (AFIH) and severe acute respiratory illness (SARI)]. The District Surveillance Unit (DSU) verified these signals, flagged verified signals as events, and conducted a risk assessment to determine if the events were outbreaks.
Results
From May to December 2023, data from 3294 (73%) of 4512 AFI patients were analysed using the EBS algorithm. Of the 88 signals identified, 67 (76%) were due to SARI, 9 (10.3%) were due to AES, and 9 (9%) were due to AFIR. Ten signals were verified as events, of which nine were classified as outbreaks (dengue-1, H1N1-3, H3N2-1, H1N1 and H3N2 - 1, H1N1 and SARS-COV2 – 1, no pathogen detected– 2). Five outbreaks were not detected by the existing indicator-based surveillance (IBS).
Interpretation & conclusions
EBS pilot in private health facilities complemented the IBS system by early detecting outbreaks. This EBS model has the potential for implementation in other districts, especially in districts at higher risk of zoonotic spillover.
Keywords
Emerging communicable diseases
India
public health surveillance
sentinel surveillance
virus diseases
The International Health Regulations (IHR) 2005 of the World Health Organization (WHO) mandates that countries establish early warning and response (EWAR) systems that can promptly detect, report, and mitigate emerging infectious disease threats1. An optimal EWAR system utilises both indicator-based surveillance (IBS) and event-based surveillance (EBS) to effectively detect outbreaks2. IBS involves collecting structured surveillance data, establishing specific indicators and identifying concerning trends or patterns. An EBS, in contrast, is ‘an organised collection, monitoring, assessment, and interpretation of unstructured, ad-hoc information regarding health events or risks, which may represent an acute risk to human health’3. EBS can be established at the healthcare facility or community levels. A comprehensive review of 13 diverse EBS systems revealed that both health facility and community-based EBS offer valuable insights that can significantly enhance the early warning capabilities of national surveillance systems4. A community-based EBS project implemented in Tamil Nadu demonstrated the added value of such a system to the existing surveillance system in terms of detecting early warning signals5. An EBS established in Kenya, 2020 using an electronic reporting tool identified 8790 signals and 3002 events across both community and health facility sites over 15 months6. Another EBS pilot in Vietnam resulted in early detection and reporting of outbreaks, improved collaboration between the healthcare facilities and preventive sectors of the ministry, and increased community participation in surveillance and reporting7. Previously, EBS was utilised in mass gatherings in India for early detection of outbreaks8,9.
In the Indian State of Kerala, the Integrated Disease Surveillance Programme (IDSP) primarily functions as an IBS. The diseases/syndromes under surveillance are reported using standard case definitions from the communities, hospitals, and laboratories using syndromic (S), presumptive (P), and laboratory (L) surveillance forms, respectively, and analysed weekly for disease trends10. EBS is integrated into routine surveillance through the use of rumour registers maintained at the peripheral health institutions and dedicated media scanning units at the central and State levels11.
Approximately 70 per cent of the population in Kerala seeks care in private hospitals. However, the detection of outbreak and disease clusters primarily relies on surveillance data generated through public sector facilities12. This presents an opportunity to strengthen the disease surveillance system by leveraging data from private hospitals. For instance, recent outbreaks of Nipah, Monkeypox, and Zika were all initially reported from private hospitals13-15, highlighting their critical role in the early detection of disease outbreaks. Therefore, establishing an EBS in private hospitals could augment epidemic intelligence to identify infectious disease threats in the State. We piloted an EBS model in selected private hospitals in Kasaragod district to assess its added value in providing early warning signals for potential disease outbreaks.
Materials & Methods
This study was conducted by Inetgrated Disease Surveillance Programme, Kasaragod, Kerala and ICMR-National Institute of Epidemiology, Chennai. The study was approved by the Institutional Human Ethics Committee of Government Medical College, Pariyaram, Kannur, Kerala. All participants provided written informed consent, assent, or parental consent as appropriate. For patients unable to provide consent due to altered mental status, we obtained consent from a family member or legally acceptable representative.
Study setting
Kerala, the southernmost State of India, has 14 administrative districts and a population of 35 million. With over 1.8 million international passengers arriving annually through the four international airports, the State faces a heightened risk of novel pathogens entering. Kerala has experienced frequent outbreaks of infectious diseases, including West Nile Virus, Kyasanur Forest Disease, Nipah, Zika, Covid-19 and MPox13-18.
We piloted the EBS system in Kasaragod, a northern district of Kerala with a population of 1.5 million, between May and December 2023. The district is characterised by local communities living close to the Western Ghats, a densely forested mountain range, and a significant proportion of young and middle-aged people working abroad. We selected six private hospitals in the district with a minimum of 60 inpatients, five intensive care beds and the highest inpatient admissions in the preceding year.
The event-based surveillance (EBS) pathway
Our hospital-based EBS focused on monitoring four syndromes: severe acute respiratory illness (SARI), acute encephalitis syndrome (AES), acute febrile illness with haemorrhage (AFIH) and acute febrile illness with rash (AFIR). These syndromes cover the typical manifestations of many emerging infectious diseases. Standard case definitions to define the syndromes19-21 were used (Box 1). Following two rounds of detailed stakeholder discussions involving physicians, pulmonologists, intensivists, and public health experts in the State, we finalized seven signals for monitoring through the EBS (Box 2).
We routinely collected data from all in-patients with acute febrile illness (AFI) with a documented temperature of ≥38°C or a history of fever within the last 10 days from the internal medicine, pulmonary medicine, obstetrics and gynaecology, paediatrics, and intensive care units of the selected hospitals. Data on demographics, initial clinical features, and a provisional diagnosis of the admitted AFI patients were extracted daily in a standardised case reporting format from the clinical records by trained clinical nurses using an electronic tablet. Once entered into a database, we used an algorithm to analyse the database to identify signals automatically. If any of the signals (Box 1) were detected, the information was emailed to the District Surveillance Unit, study hospital, and principal investigator and displayed on the dashboard automatically by the software. The epidemiologist at the DSU of IDSP verified the signals by reviewing the EBS data and gathering more information from the field through field workers (health inspector and public health nurse) of the concerned wards. A verified signal was called as an event. Once flagged as an event, the DSU did the risk assessment to classify the events as cluster/outbreak and initiated response measures as per the standard operating procedures of the IDSP22. The EBS pathway is illustrated in figure 1.
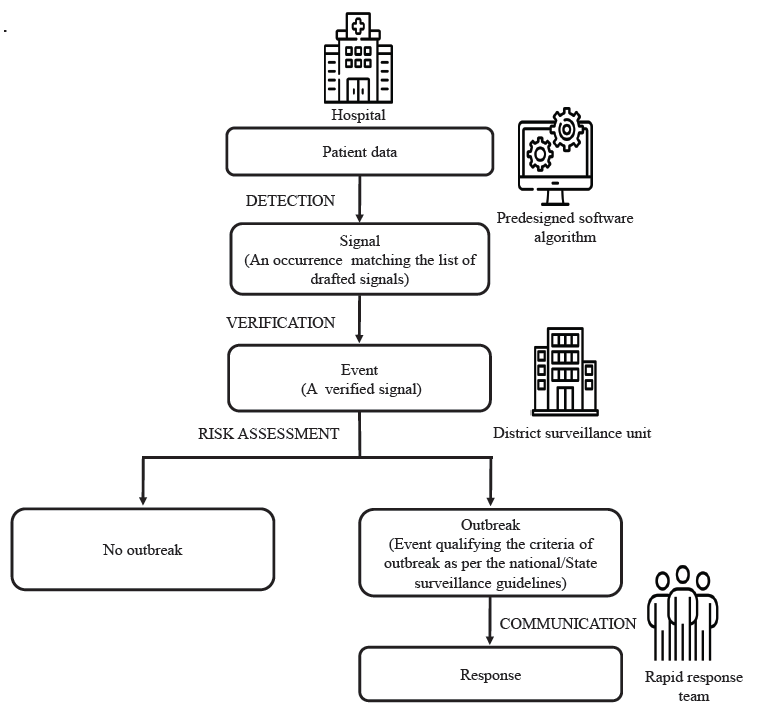
- The event-based surveillance (EBS pathway). Source: Open-source icons downloaded from Freepik (
https://www.freepik.com
).
A laboratory testing algorithm was also developed for testing clinical samples when an event was detected through EBS. The laboratory testing algorithm was adapted from the guidelines of the Viral Research and Diagnostic Laboratory Network (VRDLN) of India23. Supplementary table I shows the various pathogens tested when an event was detected.
The EBS platform
A web-based signal detection platform was developed. The platform had two portals: a data entry portal and an admin portal. The data entry portal was designed for hospitals to upload patient information using unique login credentials in a standardised case reporting format (CRF). The admin portal was developed for the monitoring by the DSU staff and the principal investigator (PI). The feature-oriented platform could perform fully scripted automated signal detection, signal visualisation, event updating, and email-based alerting of the hospitals, DSU, and PI.
Implementation of the EBS
We conducted eight meetings with stakeholders (two each with State health authorities, district health authorities, the DSU team, participating institute administrators, and medical officers). We obtained the necessary approvals to implement the project. A consensus was achieved during discussions on the signals and implementation plan, and suggestions provided by the State and district teams were incorporated into the final software. Once we developed the software and dashboard, we validated the system using pilot data to identify whether the signals were generated as per our algorithm. We made necessary alterations to ensure the system identified signals as intended.
Once the portal was validated and operational, each participating hospital nominated two clinical nurses working in the hospital for this project. We trained 12 clinical nurses from these six hospitals using hands-on data entry into the portal through our electronic tablet. Data collection was started on May 23, 2023, from all six hospitals.
Evaluation of EBS performance
To assess the performance of EBS, we calculated positive predictive values (PPV) of the system in detecting events (Number of signals verified as events/ Total number of signals) and outbreaks (Number of events confirmed as outbreaks / Total number of events). We also calculated the timeliness of the EBS by comparing the time taken to identify the event in the EBS and confirmation of the outbreak in IBS.
Quality assurance
We used electronic case report forms with built-in validation checks to reduce data entry errors. These included automated prompts and range checks to flag inconsistent or out-of-range entries in real-time. We also arranged for the data collectors to cross-verify details with the treating physician when in doubt. The investigators used a social media group for quick query resolution posted by the clinical nurses. The investigators periodically monitored the database for timeliness and completeness of entered data. We trained the district surveillance teams and field-level healthcare workers on responding to each of these signals and ensured that the laboratory sample collection logistics were in place.
Results
Enrolment of patients
The EBS was piloted during May 23, 2023, through December 23, 2023. During the pilot, we collected data from 3294 (73%) of the 4512 patients hospitalised with febrile illness in the six hospitals. A total of 894 (19.8%) patients did not consent to participate in the study, 212 (4.7%) were missed by the data collectors during the initial phase, and 112 (2.5%) were referred to another centre before data collection. The patients enrolled in the EBS were from 40 of 41 local self-government units (LSGU) in the district. The median (IQR) age was 20 (5-54) yr, with an almost equal sex distribution. The most reported comorbid conditions among patients over 30 yr were hypertension (n=463, 34%) and diabetes mellitus (n=350, 26%; Table I).
Characteristics | n | (%) |
---|---|---|
Age group (yr) | ||
Under 5 | 791 | 24 |
5-17 | 775 | 23.5 |
18-45 | 716 | 21.7 |
46-70 | 700 | 21.3 |
Above 70 | 312 | 9.5 |
Sex | ||
Male | 1683 | 51.1 |
Female | 1599 | 48.5 |
Others | 12 | 0.4 |
Admitted department | ||
Internal medicine | 1738 | 53 |
Paediatrics | 1396 | 42 |
Intensive care unit | 86 | 2.6 |
Pulmonary medicine | 60 | 2 |
Others | 14 | 0.4 |
Occupation | ||
No job | 2047 | 62 |
Student | 734 | 22.3 |
Other job | 400 | 12.2 |
Farming | 88 | 2.7 |
Livestock, poultry, pig farming | 15 | 0.5 |
Healthcare worker | 10 | 0.3 |
Smoking | ||
Current smoker | 75 | 2.3 |
Ex-smoker | 161 | 4.9 |
Never smoker | 3058 | 92.8 |
Alcohol consumption | ||
Current drinker | 123 | 3.7 |
Ex-drinker | 151 | 4.6 |
Never drinker | 3020 | 91.7 |
H/O travel outside the district in the last 14 days | 141 | 4.3 |
Comorbidities in patients above 30 yr (n=1367) | ||
Hypertension | 463 | 34 |
Diabetes mellitus | 350 | 25.6 |
Coronary artery disease | 67 | 5 |
Chronic kidney disease | 36 | 2.6 |
Chronic liver disease | 25 | 1.8 |
Chronic neurological conditions | 15 | 1.1 |
Malignancy | 5 | 0.4 |
Treatment outcome | ||
Recovered | 2989 | 90.7 |
Died | 81 | 2.5 |
Referred to another facility | 224 | 6.8 |
Description of signals
We identified 88 signals during the implementation of EBS. Figure 2 shows the timeline of signals and events. At least one signal was detected every week except for epi-weeks 21, 22, 26, and 51. Epi-week 33 detected the largest number of signals (n=12, 14%). Most signals were due to SARI (n=67, 76%), followed by AES (n=9, 10%) and AFIR (n=8, 9%). In addition, three healthcare worker signals were detected on the 23rd, 24th, and 28th epi-week, and one signal due to a death on epi-week 24 (Table II and Fig. 2). No AFIH signals were detected during the study period. Signals were detected in 28 (68%) LSGUs (Fig. 3). About one-fourth of the signals (n=20, 23%) were from three urban LSGUs in the district. Twenty-eight (32%) signals were detected from the four rural LSGUs, which were adjacent to these urban areas.
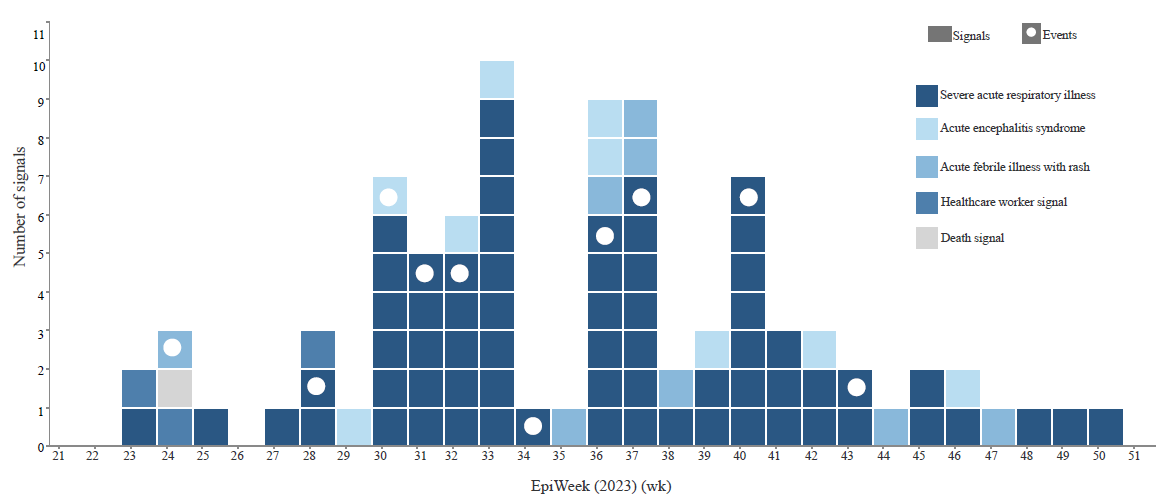
- Timeline of signal and events detected through the EBS May-December 2023.
Signal type | n | (%) |
---|---|---|
SARI signal | 67 | 76 |
AES signal | 9 | 10.3 |
AFIR signal | 8 | 9 |
Healthcare worker signal | 3 | 3.5 |
Death signal | 1 | 1.2 |
SARI, severe acute respiratory illness; AES, acute encephalitis syndrome; AFIR, acute febrile illness with rash
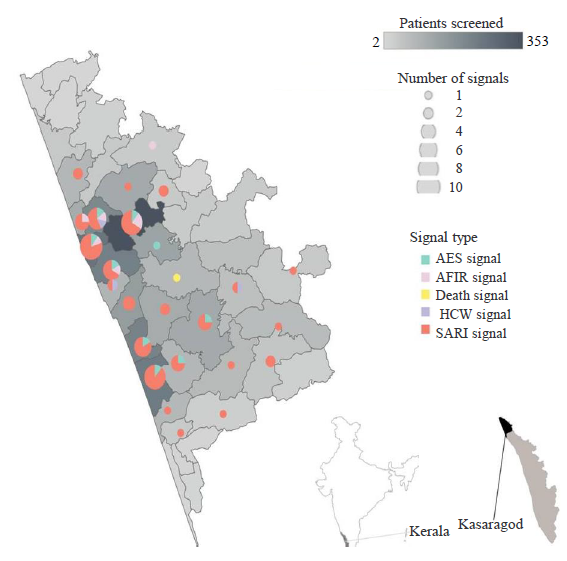
- Residence of patients who triggered EBS signals, by local self-government unit, and signal triggered. Source: Map created with QGIS using open-source shape file of the district available with the district administration.
Event identification and investigation of outbreaks/clusters
All 88 signals were investigated by the district surveillance unit: of them, 10 signals flagged as ‘events’ following verification, and the rest were discarded. The reasons for discarding the signals are provided in supplementary table II. Nine of the events were classified as outbreaks based on risk assessment (Table III). Among the 10 events identified, eight were SARI, and one each was an AFIR and AES event. Three of the eight SARI events were due to influenza A(H1N1), and one was due to influenza A(H3N2). In two events, mixed infections (influenza A(H1N1) and influenza A(H3N2), as well as influenza A(H1N1) and SARS-CoV2, were identified. No pathogen could be isolated in two SARI events. The AFIR event was due to dengue. No pathogens could be detected for the AES event (Table III). The investigation of these events resulted in declaring nine events as outbreaks and identified 305 case-patients during field investigation. In one of the SARI events classified as an outbreak, one death was reported with a case fatality ratio of 4.5 per cent (1/22) (Table III).
Event type | Date of verification of signal as an event in EBS | Date of reporting of outbreak/cluster in IBS | Case patients | Pathogen detected |
---|---|---|---|---|
AFIR* | 14/06/2023 | 19/06/2023 | 11 | DENV1, DENV3 |
SARI* | 13/07/2023 | 15/07/2023 | 16 | H1N1 |
AES | 30/07/2023 | 05/08/2023 | 4 | Unknown |
SARI* | 31/07/2023 | 06/08/2023 | 22 (including 1 death) | H1N1, SARSCoV2 |
SARI* | 12/08/2023 | Not detected | 8 | Unknown |
SARI* | 25/08/2023 | Not detected | 14 | H1N1 |
SARI* | 09/09/2023 | 14/09/2023 | 202 | H1N1, H3N2 |
SARI* | 12/09/2023 | Not detected | 6 | H1N1 |
SARI* | 07/10/2023 | Not detected | 8 | Unknown |
SARI* | 08/11/2023 | Not detected | 14 | H3N2 |
During the pilot period, EBS detected five events (4 SARI and 1AFIR) that were not identified by the routine IBS. Additionally, five events (1 dengue, 1 AES, and 3 H1N1 events) were detected through both IBS and EBS.
Performance indicators of EBS
The positive predictive value (PPV)for detecting events was 11.4 per cent (10/88), and the PPV for events confirmed as outbreaks was 90 per cent (9/10). The median time interval between identifying events in EBS and confirming outbreaks in IBS was 5 (2-6) days.
Discussion
We piloted an EBS at six private hospitals in Kasaragod, Kerala. During the seven months of surveillance, 88 signals were identified, confirming 10 events, of which nine were outbreaks. Our EBS detected five events not reported to the routine IBS system, prompting investigations and implementation of appropriate measures to control the outbreak.
Community-based and facility-based EBS has been successfully used for EWAR systems in many countries, especially in regions with weak surveillance infrastructure24-28. For example, a combination of IBS and EBS has been established in government hospitals in Bangladesh for Nipah surveillance25. In Vietnam, the establishment of EBS at community and healthcare facilities has resulted in earlier detection of outbreaks, better collaboration between facilities, and improved community engagement for surveillance24. The IDSP has envisaged event-based reporting tools such as rumour reporting, media surveillance, toll-free numbers, and community-based surveillance to increase the sensitivity of its routine IBS. However, it has not been uniformly implemented across the country29. The integrated health information platform (IHIP) system has features for reporting events. Still, it lacks the automated facility to detect signals/events from the patient line list, highlighting the need for facility-based EBS.
Recent outbreaks of Nipah and Zika viruses in Kerala were first reported from private hospitals13,14. Our study demonstrated the feasibility of engaging private sector hospitals in EBS and its role in enhancing the surveillance to detect disease clusters in the State. Such a model can significantly complement existing surveillance for emerging and re-emerging infectious disease threats. Although our EBS was established in only six hospitals in the district, we could screen patients seeking care from all but one of the 41 administrative units in the district. This may be due to the peculiar socio-demographic-geological aspects of the district, where the leading hospitals are concentrated in the two major townships, and there are very few in-patient care facilities in semi-urban and rural settings. Our EBS focused mainly on four syndromes: SARI, AES, AFIR, and AFIH. However, the same platform can be adapted to include additional syndromes/conditions of public health importance based on the local context. Establishing electronic health records at all treating facilities and automated linkage to the EBS can significantly improve the system’s efficiency. A major gap in outbreak investigation is the lack of training for the rapid response team at the field level30. By training the field staff, we ensured a swift and quality investigation from the rapid response team (RRT) to the identified events. We also tracked all outbreaks detected through EBS until the completion of the outbreak cycle.
EBS could detect outbreaks earlier than IBS, allowing more time to initiate the response. In highly communicable disease outbreaks such as Nipah, even a single day could play a significant role in reducing the transmission. EBS demonstrated earlier detection, identifying five additional health events with a median lead time of five days compared to IBS. This advantage stems from EBS’s reliance on real-time, unstructured data from clinical observations, which enable prompt responses to emerging threats. In contrast, IBS depends on structured, aggregated data from standardised reporting forms, which can delay event recognition. However, EBS requires continuous monitoring, trained personnel, and a robust reporting framework, which may strain resources, especially in low-resource settings. Conversely, IBS benefits from established protocols and automated reporting in many health systems but may miss atypical or emerging events.
The cornerstone of our EBS project was its integration with the existing national communicable disease surveillance system, IDSP, and its utilisation of VRDL’s laboratory services. We propose the following strategies to address resource constraints while scaling up the EBS. Task-shifting to less specialised healthcare workers, supported by targeted training and supervision, can help reduce dependence on highly trained clinical nurses. Leveraging the use of mobile apps for real-time data collection, and integrating EBS tasks into routine hospital workflows, and securing dedicated funding through public-private partnerships can ensure sustainability.
While our study demonstrates the feasibility of implementing EBS in six sentinel hospitals within a single district, we acknowledge that the limited geographic and institutional scope may constrain the generalizability of our findings. However, this proof-of-concept highlights the essential considerations for scaling the EBS model to diverse healthcare settings. To enhance scalability, future implementations should tailor the selection of sentinel hospitals to reflect local healthcare delivery structures, incorporating both secondary and tertiary care facilities across urban and rural regions. Establishing robust data-sharing protocols with public health authorities and integrating EBS with existing surveillance systems will be critical to ensure seamless data flow and timely action. Additionally, addressing resource requirements, including staffing and technological infrastructure, will be essential, particularly in resource-constrained settings. Finally, fostering private sector engagement through supportive policies and governance frameworks will play a key role in scaling the model effectively. These strategies can help extend the utility of the EBS model across varied contexts, strengthening its impact on public health surveillance.
Challenges in implementing the EBS
One of the major challenges we faced was onboarding private-sector hospitals. The hospitals were initially apprehensive, particularly about the perceived increased burden for staff. However, they appreciated the simplicity of the portal, which required minimal time and resource commitment. The key factors facilitating their participation in the pilot included a user-friendly data entry platform, access to advanced laboratory testing facilities for their patients in case of signals/events, and the added utility of the EBS to public health surveillance. Because our EBS was fully automated, the data entry quality was crucial for project success. To ensure data quality, we trained and utilised clinical nurses from the enrolled hospitals as data collectors with compensation for their time. This ensured the timely collection of precise data from the patient’s clinical records. Another challenge we faced during our pilot was limited training for field-level workers in outbreak response. To address this, we conducted training sessions and provided them with standard operating procedures (SOPs) to respond to outbreaks. There was also a gap in obtaining and testing samples from outbreaks detected. As part of the project, we followed the sample testing algorithms of the VRDL and utilised their logistics for sample collection, storage, transport, and testing of various viral pathogens. The core component of our project was its linkage with the district surveillance unit of IDSP. Achieving this required significant efforts and multiple deliberations with the State and district health authorities to obtain the necessary permissions. This integration was vital for ensuring that the system complemented existing surveillance mechanisms, and facilitated prompt verification of the signals and initiating public health responses for the identified events.
Our study has certain limitations. First, about 20 per cent of eligible patients refused consenting to participate in the study. This may be because the clinical nurses could not clearly explain the study to the patients, particularly in the early data-collection phase. This was addressed by training the nurses about obtaining written informed consent. As our study focused on identifying clustering of cases by time and location, we could have detected more signals if the non-response in the study was lower. Second, we selected hospitals for the pilot based on the patient load and functional intensive care units (ICUs). We assumed that patients with severe manifestations, including those with SARI, AES, and AFIH syndromes, were more likely to get admitted to these secondary/tertiary care hospitals. However, the selected hospitals might not represent the entire private sector in the district. Another limitation of the pilot was access to metagenomic next-generation sequencing (NGS) for events where the aetiology could not be identified based on serological and molecular tests. Incorporating NGS capabilities in future iterations of the EBS system could greatly enhance the ability to identify novel pathogens, facilitating the early detection of emerging infectious disease threats. Since we did not have information from the IBS portal on the total number of outbreaks in the catchment area of the study hospitals, we were unable to calculate sensitivity. However, future evaluations of full-scale EBS implementation may consider collecting data about additional attributes such as sensitivity and usefulness31.
Despite these limitations, the findings of our pilot indicated that establishing EBS at private-sector hospitals in Kerala complimented the IBS by early detecting outbreaks. Given its added value in strengthening disease surveillance, the IDSP may consider implementing this model in other districts, especially those at higher risk of zoonotic spillover.
Financial support & sponsorship
Abbott Pandemic Defence Coalition (APDC)-Field Epidemiology Training Program (FETP) Fellowship 2022-2023.Task Force for Global Health, Inc. Contract #6417/3913, funded through a grant from Abbott Laboratories for Medical Education or Programs.
Conflicts of Interest
None.
Use of Artificial Intelligence (AI)-Assisted Technology for manuscript preparation
The authors confirm that there was no use of AI-assisted technology for assisting in the writing of the manuscript and no images were manipulated using AI.
References
- Global health. International health regulations (IHR). Available from: https://www.cdc.gov/globalhealth/healthprotection/ghs/ihr/index.html, accessed on July 10, 2024.
- Early detection, assessment and response to acute public health events: implementation of early warning and response with a focus on event-based surveillance: Interim version. Available from: https://apps.who.int/iris/handle/10665/112667, accessed on December 1, 2022.
- The importance of event-based surveillance for preparedness and response in future respiratory pandemics. J Glob Health. 2021;11:03098.
- [CrossRef] [PubMed] [PubMed Central] [Google Scholar]
- Event-based surveillance at health facility and community level in low-income and middle-income countries: A systematic review. BMJ Glob Health. 2019;4:e001878.
- [CrossRef] [PubMed] [PubMed Central] [Google Scholar]
- Lessons learnt in implementing a pilot community event-based surveillance system in Tiruvallur district, Tamil Nadu, India. Glob Security: Health, Sci Policy. 2020;5:111-20.
- [CrossRef] [Google Scholar]
- Kenya’s experience implementing event-based surveillance during the COVID-19 pandemic. BMJ Glob Health. 2023;8:e013736.
- [CrossRef] [PubMed] [PubMed Central] [Google Scholar]
- Event-based surveillance at community and healthcare facilities, Vietnam, 2016-2017. Emerg Infect Dis. 2018;24:1649-58.
- [CrossRef] [PubMed] [Google Scholar]
- Disease surveillance during a large religious mass gathering in India: The Prayagraj Kumbh 2019 experience. Int J Infect Dis. 2020;101:167-73.
- [CrossRef] [PubMed] [PubMed Central] [Google Scholar]
- Syndromic surveillance system during mass gathering of Panchkroshi Yatra festival, Ujjain, Madhya Pradesh, India. New Microbes New Infect. 2023;52:101097.
- [CrossRef] [PubMed] [PubMed Central] [Google Scholar]
- Home: Integrated disease surveillance programme (IDSP). [homepage on the Internet; updated 2025 January 27]. Available from: https://www.idsp.mohfw.gov.in/, accessed on January 27, 2025.
- Media scanning as a supplemental tool to disease outbreak reporting under IDSP-India an event based surveillance under Integrated disease surveillance project (IDSP) J Commun Dis. 2009;41:257-62.
- [Google Scholar]
- Public and private healthcare Institutions: Preference and expenditure pattern. Working paper no. 1; 2017. Available from: https://www.academia.edu/download/53775910/Public_and_Private_Healthcare_Institutions_Preference_and_Expenditure_Pattern.pdf, accessed on March 24, 2024.
- Outbreak investigation of nipah virus disease in Kerala, India, 2018. J Infect Dis. 2019;219:1867-78.
- [CrossRef] [PubMed] [Google Scholar]
- Detection of Zika virus disease in Thiruvananthapuram, Kerala, India 2021 during the second wave of COVID-19 pandemic. J Med Virol. 2022;94:2346-9.
- [CrossRef] [PubMed] [PubMed Central] [Google Scholar]
- First two cases of Monkeypox virus infection in travellers returned from UAE to India, July 2022. J Infect. 2022;85:e145-8.
- [CrossRef] [PubMed] [PubMed Central] [Google Scholar]
- West Nile encephalitis outbreak in Kerala, India, 2011. J Clin Virol. 2014;61:152-5.
- [CrossRef] [PubMed] [Google Scholar]
- An outbreak of Kyasanur forest disease in the Wayanad and Malappuram districts of Kerala, India. Ticks Tick Borne Dis. 2017;8:25-30.
- [CrossRef] [PubMed] [Google Scholar]
- A confirmed case of COVID-19 among the first three from Kerala, India. Indian J Med Res. 2020;151:493-4.
- [CrossRef] [PubMed] [PubMed Central] [Google Scholar]
- WHO Surveillance case definitions for ILI and SARI. Available from: https://www.who.int/teams/global-influenza-programme/surveillance-and-monitoring/case-definitions-for-ili-and-sari, accessed on February 9, 2024.
- Viral hemorrhagic fever (VHF) 2022 case definition. Available from: https://ndc.services.cdc.gov/case-definitions/viral-hemorrhagic-fever-2022/, accessed on February 9, 2024.
- A cohort study to assess the new WHO Japanese encephalitis surveillance standards. Bull World Health Organ. 2008;86:178-86.
- [CrossRef] [PubMed] [PubMed Central] [Google Scholar]
- Manuals & guidelines: Integrated disease surveillance programme (IDSP). Available from: https://idsp.mohfw.gov.in/index1.php?lang=1&level=1&sublinkid=5821&lid=3765, accessed on March 24, 2024.
- Virus research and diagnostic laboratory (VRDL) ICMR-NICED. Available from: https://www.niced.org.in/niced/VRDL-ICMR-NICED.htm, accessed on March 24, 2024.
- Event-based surveillance at community and healthcare facilities, Vietnam, 2016-2017. Emerg Infect Dis. 2018;24:1649-58.
- [CrossRef] [PubMed] [Google Scholar]
- Integrated cluster- and case-based surveillance for detecting stage III zoonotic pathogens: An example of Nipah virus surveillance in Bangladesh. Epidemiol Infect. 2015;143:1922-30.
- [CrossRef] [PubMed] [PubMed Central] [Google Scholar]
- Assessment of community event-based surveillance for ebola virus disease, Sierra Leone, 2015. Emerg Infect Dis. 2016;22:1431-7.
- [CrossRef] [PubMed] [PubMed Central] [Google Scholar]
- Event-based surveillance in north-western Ethiopia: Experience and lessons learnt in the field. Western Pac Surveill Response J. 2015;6:22-7.
- [CrossRef] [PubMed] [PubMed Central] [Google Scholar]
- Establishing event-based surveillance system in Nigeria: A complementary information generating platform for improved public health performance, 2016. Pan Afr Med J. 2022;42:63.
- [CrossRef] [PubMed] [PubMed Central] [Google Scholar]
- Communicable disease outbreak detection by using supplementary tools to conventional surveillance methods under integrated disease surveillance project (IDSP), India. J Commun Dis. 2009;41:149-59.
- [PubMed] [Google Scholar]
- Evaluation through outbreak simulation exercise points to the need for considerable improvement in the capacity of peripheral health workers for outbreak detection and response, South India, 2018. J Family Med Prim Care. 2021;10:1587-91.
- [CrossRef] [PubMed] [PubMed Central] [Google Scholar]
- An indicator framework for the monitoring and evaluation of event-based surveillance systems. Lancet Glob Health. 2024;12:e707-11.
- [CrossRef] [PubMed] [PubMed Central] [Google Scholar]